- CFA Exams
- 2025 Level II
- Topic 1. Quantitative Methods
- Learning Module 7. Big Data Projects
- Subject 3. Data Exploration Objectives and Methods
Why should I choose AnalystNotes?
AnalystNotes specializes in helping candidates pass. Period.
Subject 3. Data Exploration Objectives and Methods PDF Download
Data exploration encompasses three tasks:
-
Exploratory data analysis. You do this by taking a broad look at patterns, trends, outliers, unexpected results, and so on in your existing data, using visual and quantitative methods to get a sense of the story this tells. The ability to find insight and be able to communicate it effectively in an organization is fuelled with strong EDA capabilities.
- Feature selection. It is the process of reducing input features to the most informative ones for use in model construction.
- Feature engineering is the process of using domain knowledge of the data to create features that make machine learning algorithms work.
Structured Data
- Exploratory data analysis. Visualizations are histograms, bar charts, box plots and density plots for one-dimensional data, scatterplots and line graphs for two-dimensional data, stacked bar, line charts and multiple box plots for multivariate data. Descriptive statistics such as mean, max, standard deviations, correlation matrix can also be used to summarize data.
- Feature selection. You need not to use every feature at your disposal for creating an algorithm. You can assist your algorithm by feeding in only those features that are really important. It reduces overfitting. It is a methodical and iterative process.
- Feature engineering techniques systemically alter, decompose, or combine existing features to produce more meaningful features.
Unstructured Data: Text Exploration
- Exploratory data analysis. You can quickly perform these tasks (tokenize text, remove stop words, count text pairs) to gain practically useful insights from the text data. You can then visualize this with tools like bar charts and word clouds.
- Feature selection methods used for text data include term frequency, document frequency, chi-square test, and a mutual information measure.
- Feature engineering for text data includes converting numbers into tokens, creating n-grams, and using name entity recognition and parts of speech to engineer new feature variables.
User Contributed Comments 0
You need to log in first to add your comment.
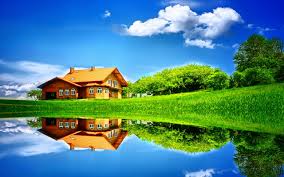
I used your notes and passed ... highly recommended!
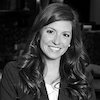
Lauren
My Own Flashcard
No flashcard found. Add a private flashcard for the subject.
Add