- CFA Exams
- 2025 Level II
- Topic 1. Quantitative Methods
- Learning Module 3. Model Misspecification
- Subject 1. Model Misspecification
Seeing is believing!
Before you order, simply sign up for a free user account and in seconds you'll be experiencing the best in CFA exam preparation.
Subject 1. Model Misspecification PDF Download
Model specification refers to the set of variables included in the regression and the regression equation's functional form.
Principles of model specification:
- The model should be grounded in cogent economic reasoning.
- The functional form chosen for the variables in the regression should be appropriate given the nature of the variables.
- The model should be parsimonious.
- The model should be examined for violations of regression assumptions before being accepted.
- The model should be tested and be found useful out of sample before being accepted.
If a regression is misspecified, then statistical inference using OLS is invalid and the estimated regression coefficients may be inconsistent.
Assuming a model has the correct functional form, when in fact it does not, is one example of misspecification. There are several ways this assumption may be violated:
- omitted variables
- inappropriate form of variables
- inappropriate variable scaling
- inappropriate data pooling
Another type of misspecification occurs when independent variables are correlated with the error term. This is a violation of Regression Assumption 4, that the error term has a mean of 0, and causes the estimated regression coefficients to be biased and inconsistent. Three common problems that create this type of time-series misspecification are:
- including lagged dependent variables as independent variables in regressions with serially correlated errors.
- including a function of dependent variables as an independent variable, sometimes as a result of the incorrect dating of variables; and
- independent variables that are measured with error.
Avoiding Model Misspecification
1. Transforming Non-linear Variables to a Linear Form
Non-linear relationships can exist between variables. However, most statistical models assume linearity. As such, it is often necessary to transform non-linear variables into a linear form before modeling them. This can be done using log-based transformations or other methods.
Log-based transformations help to normalize the data and make it easier to model. In addition, they can help to improve the interpretability of the results.
2. Avoiding Independent Variables that are Mathematical Functions of Dependent Variables
In some cases, an independent variable may be a mathematical function of the dependent variable. For example, the dependent variable may be total revenue, and the independent variable may be sales price per unit. In this case, the sales price per unit is a function of total revenue (i.e., it is derived from total revenue). As such, it should not be used as an independent variable in the model because doing so would violate the assumption of no perfect multicollinearity.
3. Omitting Spurious Independent Variables
Spurious independent variables are not related to the dependent variable but are included in the model due to chance or other factors. For example, assume that two variables are highly correlated with each other (i.e., they are perfectly multicollinear). In that case, one of them may be spuriously included in the model even though it is not related to the dependent variable. Whenever possible, it is a good idea to check for multicollinearity before building the model.
4. Validate Model Estimations Out-of-Sample
One way to avoid model misspecification is to validate the model estimations out-of-sample. This implies testing the model on data that was not used to estimate the model in the first place. If the model performs well out-of-sample, we can be more confident that it is correctly specified.
5. Use Good Samples When Collecting Data
Another way to avoid model misspecification is to use good samples when collecting data. This means data should be collected from a representative sample of the population. If we do not have a good sample, our results may be inaccurate.
6. Check for Violations of Linear Regression Assumptions Using Diagnostic Tests
Checking for violations of linear regression assumptions using diagnostic tests can help determine if the data meet the assumptions necessary for linear regression. If the assumptions are not met, then the model may be misspecified.
User Contributed Comments 0
You need to log in first to add your comment.
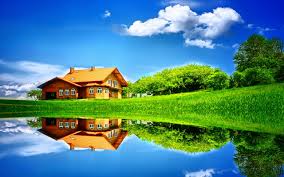
I passed! I did not get a chance to tell you before the exam - but your site was excellent. I will definitely take it next year for Level II.
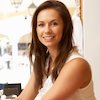
Tamara Schultz
My Own Flashcard
No flashcard found. Add a private flashcard for the subject.
Add