- CFA Exams
- 2025 Level II
- Topic 1. Quantitative Methods
- Learning Module 3. Model Misspecification
- Subject 4. Multicollinearity
Why should I choose AnalystNotes?
Simply put: AnalystNotes offers the best value and the best product available to help you pass your exams.
Subject 4. Multicollinearity PDF Download
Multicollinearity occurs when two or more independent variables are highly (but not perfectly) correlated across observations, even though the regression equation seems to fit rather well. With Multicollinearity, the regression coefficients may not be individually statistically significant even when the overall regression is significant as judged by the F-statistic.
Multicollinearity results in inflated standard errors and reduced t-statistics.
The existence of multicollinearity causes great problems in the field of statistical inference, as correcting for this form of estimation bias is often not possible. One way to check for multicollinearity is to find the sample correlation coefficients between all pairs of potential explanatory variables in the model. When the sample correlation coefficient between two variables is large in absolute value, it may be difficult to separate the effects of these two variables on the dependent variable Y.
For example, a multivariate regression is defined by three independent variables. The t-statistics for these three independent variables, as well as the intercept, are as follows:
- t-intercept: 0.87
- t-independent variable one: -0.26
- t-independent variable two: 0.32
- t-independent variable three: -0.47
- R2: 0.95
When α is equal to 0.05, the critical value for the t-statistic is 1.735.
The regression profiled in this example appears to suffer from multicollinearity bias. This is indicated by the low t-statistics for the regression coefficients and the suspiciously high R2. These two indicators occurring in conjunction should raise red flags as a possible indication of multicollinearity bias.
Correcting Multicollinearity
There are a few methods of correcting multicollinearity:
Reducing the number of predictor variables in the model by excluding some of them or combining two or more correlated predictors into one. This is often done by conducting feature selection techniques, such as forward selection, backward selection and stepwise regression.
Regularization methods such as ridge regression and lasso regression. These reduce the magnitude of coefficients for predictors that are highly correlated with each other, thus penalizing large coefficient values associated with these predictors and reducing their influence on the model.
Decorrelation methods which involve transforming the data so that predictors become uncorrelated. One popular way of doing this is Principal Component Analysis (PCA), where orthogonal components are derived from the original set of variables, with each component having uncorrelated predictors in it.
Collinearity diagnostics can also be used to identify pairs of highly correlated variables and then take appropriate action to reduce multicollinearity in those cases. This includes examining Spearman's correlation coefficients and Variance Inflation Factors (VIF).
User Contributed Comments 1
User | Comment |
---|---|
klombard | example above is good |
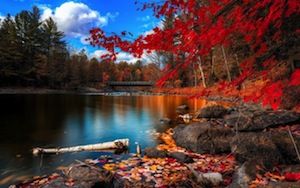
You have a wonderful website and definitely should take some credit for your members' outstanding grades.
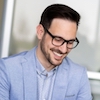
Colin Sampaleanu
My Own Flashcard
No flashcard found. Add a private flashcard for the subject.
Add