- CFA Exams
- 2023 Level I > Topic 1. Quantitative Methods > Reading 5. Sampling and Estimation
- 7. Data Snooping Bias, Sample Selection Bias, Look-Ahead Bias, and Time-Period Bias
Why should I choose AnalystNotes?
Simply put: AnalystNotes offers the best value and the best product available to help you pass your exams.
Subject 7. Data Snooping Bias, Sample Selection Bias, Look-Ahead Bias, and Time-Period Bias
There are a number of different types of bias that can creep into samples. It is important to be aware of them and have the ability to comment on their possible appearance in the data where appropriate.
Data-snooping bias is the bias in the inference drawn as a result of prying into the empirical results of others to guide your own analysis.
Finding seemingly significant but in fact spurious patterns in data is a serious problem in financial analysis. Although it afflicts all non-experimental sciences, data-snooping is particularly problematic for financial analysis because of the large number of empirical studies performed on the same datasets. Given enough time, enough attempts, and enough imagination, almost any pattern can be teased out of any dataset. In some cases, these spurious patterns are statistically small, almost unnoticeable in isolation. But because small effects in financial calculations can often lead to very large differences in investment performance, data-snooping biases can be surprisingly substantial.
For example, after examining the empirical evidence from 1986 to 2002, Professor Minard concludes that a growth investment strategy produces superior investment performance. After reading about Professor Minard's study, Monica decides to conduct research of growth versus value investing based on the same or related historical data used by Professor Minard. Monica's research is subject to data-snooping bias because, among other things, the data used by Professor Minard may be spurious.
The best way to avoid data-snooping bias is to examine new data. However, data-snooping bias is difficult to avoid because investment analysis is typically based on historical or hypothesized data.
Data-snooping bias can easily lead to data-mining bias.
Data-mining is the practice of finding forecasting models by extensive searching through databases for patterns or trading rules (i.e., repeatedly "drilling" in the same data until you find something). It has a very specific definition: continually mixing and matching the elements of a database until one "discovers" two more or more data series that are highly correlated. Data-mining also refers more generically to any of a number of practices in which data can be tortured into confessing anything.
Two signs may indicate the existence of data-mining in research findings about profitable trading strategies:
- Many of the variables actually used in the research are not reported. These terms may indicate that the researchers were searching through many unreported variables.
- There is no plausible economic theory available to explain why these strategies work.
To avoid data-mining, analysts should use out-of-sample data to test a potentially profitable trading rule. That is, analysts should test the trading rule on a data set other than the one used to establish the rule.
Sample selection bias occurs when data availability leads to certain assets being excluded from the analysis. The discrete choice has become a popular tool for assessing the value of non-market goods. Surveys used in these studies frequently suffer from large non-response numbers, which can lead to significant bias in parameter estimates and in the estimate of mean.
Survivorship bias is the most common type of sample selection bias. It occurs when studies are conducted on databases that have eliminated all companies that have ceased to exist (often due to bankruptcy). The findings from such studies most likely will be upwardly biased, since the surviving companies will look better than those that no longer exist. For example, many mutual fund databases provide historical data about only those funds that are currently in existence. As a result, funds that have ceased to exist due to closure or merger do not appear in these databases. Generally, funds that have ceased to exist have lower returns relative to the surviving funds. Therefore, the analysis of a mutual fund database with survivorship bias will overestimate the average mutual fund return because the database only includes the better-performing funds. Another example is the return data on stocks listed on an exchange, as it is subject to survivorship bias; it's difficult to collect information on delisted companies and these companies often have poor performances.
Look-ahead bias exists when studies assume that fundamental information is available when it is not. For example, researchers often assume that a person had annual earnings data in January; in reality, the data might not be available until March. This usually biases results upwards.
Time period bias occurs when a test design is based on a time period that may make the results time-period specific. Even the worst performers have months or even years in which they look wonderful. After all, stopped clocks are right twice a day. To eliminate strategies that have just been lucky, research must encompass many years. However, if the time period is too long, the fundamental economic structure may have changed during the time frame, resulting in two data sets that reflect different relationships.
Practice Question 1
What is the best way to test for data-mining bias?A. Evaluate results against out-of-sample data.
B. Examine the record of the researchers.
C. Examine the database used for bias.Correct Answer: A
The downfall of most analyses that suffer from data-mining bias is their performance with out-of-sample data. Data from the sample itself should fit well, because that is the data used in creating the rule. A better test is one based on data not used in creating it. The best type of out-of-sample data is, of course, future data - and the rule's future performance.
Practice Question 2
Symptoms of data mining include ______.I. excessive numbers of variables
II. use of certain words in the study such as "we noted" or "we noticed"
III. research with slight variations from prior studiesCorrect Answer: I, II and III
These are all indications that the researchers may have succumbed to data mining.
Practice Question 3
Data mining consists of ______.A. actively searching for new data
B. over-researching a dataset until a pattern is found
C. trying to find several datasets that support the same conclusionCorrect Answer: B
Data mining consists of over-researching a dataset until a pattern is found.
Practice Question 4
Which of the following statements is incorrect?A. Data-snooping bias can be corrected for in the dataset.
B. Data-snooping bias is influenced by previous studies.
C. The analysis of new data can prevent data-snooping bias.Correct Answer: A
Data-snooping bias cannot be corrected for in the dataset. This type of bias is not in the dataset. It is in the conclusions that we derive from the dataset. Those conclusions might be influenced by prior studies that we have used in our own research. We may conclude that our data supports the same conclusions as those studies when in actuality the patterns that we observe are due to chance.
Practice Question 5
A strategy that was based on data that suffers from look-ahead bias can seem ______.A. biased
B. unfounded
C. successfulCorrect Answer: C
Look-ahead biased data can make a strategy appear successful when in actuality the apparent success results from perfect forecasting ability.
Practice Question 6
Which of the following is not a type of sample selection bias?A. survivorship bias
B. look-ahead bias
C. time-period bias
D. collection bias Correct Answer: D
Sample selection bias includes three types of bias: survivorship bias, look-ahead bias, and time-period bias. Collection bias is not a type of sample selection bias.
Practice Question 7
One of the effects of survivorship bias is ______.A. the conclusion that average return is inversely related to price-to-book ratio
B. that it allows results, which are time-specific, to be generalized
C. that companies with high returns are under-represented in samples drawn from the populationCorrect Answer: A
According to Kothari, Shanken, and Sloan, survivorship bias induces researchers to conclude that average return is inversely related to price-to-book ratio.
Practice Question 8
The bias in the inference you draw as a result of prying into the empirical results of others to guide your own analysis is known as ______.A. survivorship bias
B. data-snooping bias
C. data-mining biasCorrect Answer: B
The bias in the inference you draw as a result of prying into the empirical results of others to guide your own analysis is known as data-snooping bias.
Practice Question 9
When data availability leads to certain assets being excluded from an analysis, we call the resulting problem ______.A. survivorship bias
B. data-snooping bias
C. sample selection biasCorrect Answer: C
Practice Question 10
What type of bias is a test design subject to if it uses information that was not available on the test date?A. look-ahead bias
B. data-mining bias
C. sample selection biasCorrect Answer: A
Practice Question 11
True or False? The best way to avoid data-snooping bias is to use data that has been examined and used by other experts.Correct Answer: FalsePractice Question 12
What is the best way to avoid data-snooping bias?A. Examine fresh data if it is available.
B. Ignore the prior research of others until after you do your own study.
C. Remove snooped data from your own analysis.Correct Answer: A
The best way to avoid data-snooping bias is to examine fresh data, if it is available. This can be difficult in investment research, as the historical record is well-documented and fresh data are only added at a slow rate.
Practice Question 13
What monthly payment is required over the next 48 months to pay off a $10,000 debt today, if interest is charged at 14% per year, compounded monthly?A. $270.30
B. $366.67
C. $116.02Correct Answer: A
On the BAII Plus, press 48 N, 14 divide 12 = I/Y, 10000 PV, 0 FV, CPT PMT. On the HP12C, press 48 n, 14 ENTER 12 divide i, 10000 PV, 0 FV, PMT. Make sure the BAII Plus has the P/Y value set to 1.
Or Annuity = 10000 / {[1 - 1/(1+0.14/12)48]/(0.14/12) x (1 + 0.14/12)} = 270.30
Practice Question 14
What is the term for the bias in your inferences resulting from excessive reliance on the empirical results of others in designing your own analysis?A. sample selection bias
B. data-mining
C. data-snoopingCorrect Answer: C
Data-snooping is the bias in your inferences resulting from excessive reliance on the empirical results of others in designing your own analysis.
Practice Question 15
An examination of the historical performance of stocks currently trading on major stock exchanges will likely suffer from ______.A. survivorship bias
B. time-period bias
C. sample selection biasCorrect Answer: A
Survivorship bias is a type of sample selection bias in which entities that dropped out of the study during the study period are excluded. Consequently, an analysis of historical performances of stocks must take into account those in existence at the beginning of the period. If they drop out during the study period, then a total loss on those shares should be factored into the historical performance of the group of stocks. By selecting only those currently trading (i.e., the survivors), we ignore the poor performance of those that dropped out.
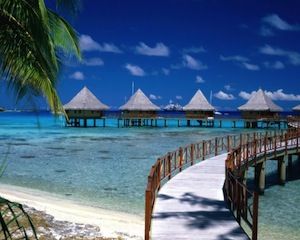
Study notes from a previous year's CFA exam:
7. Data Snooping Bias, Sample Selection Bias, Look-Ahead Bias, and Time-Period Bias