- CFA Exams
- 2025 Level II
- Topic 1. Quantitative Methods
- Learning Module 4. Extensions of Multiple Regression
- Subject 2. Using Dummy Variables in Regressions
Seeing is believing!
Before you order, simply sign up for a free user account and in seconds you'll be experiencing the best in CFA exam preparation.
Subject 2. Using Dummy Variables in Regressions PDF Download
Some observed phenomena are qualitative rather than quantitative and thus cannot be measured on a continuous scale. For example, an individual's income might depend on whether the person possesses a college degree. Dummy variables are specially constructed variables that indicate the presence or absence of some characteristics. They assume a value of 1 or 0, depending upon whether a certain characteristic is present.
An intercept dummy adds to or reduces the original intercept if a specific condition is met. When the intercept dummy is 1, the regression line shifts up or down parallel to the base regression line.
A slope dummy allows for a changing slope if a specific condition is met. When the slope dummy is 1, the slope changes to (dj + bj) × Xj, where dj is the coefficient on the dummy variable and bj is the slope of Xj in the original regression line.
Suppose the salaries of employees at a particular research institute depend on seniority (or number of years employed at the institute), whether the employee has a Ph. D. degree, and other random factors.
Suppose we express the relationship between the variables in terms of the following multiple regression model:
where
- Y = salary in dollars
- X = years in seniority
- Z = 1 if individual has a Ph. D, or 0 if individual does not have a Ph.D.
Suppose the t-th individual has seniority of Xt years and does not have a Ph.D. Thus, the variable Z assumes the value 0. The expected salary would be E(Yt | Xt, Zt = 0) = β0 + β1Xt.
A person who has a Ph.D and the same seniority of Xt years would have an expected salary of E(Yt | Xt, Zt = 1) = β0 + β1Xt + β2.
Suppose β0 is $15,000, β1 is $1,000, and β2 is $2,500. The expected salary of a non-Ph.D. with Xt years of seniority would be E(Yt | Xt, Zt = 0) = 15000 + 1000Xt. The constant β0 = $15,000 represents the starting salary, and the coefficient β1 = $1,000 represents the annual salary increment.
The expected salary of a Ph.D. with Xt years of seniority would be E(Yt | Xt, Zt = 1) = 15000 + 1000Xt + 2500. The coefficient β2 = $2,500 represents the effect of having the Ph.D as opposed to not having the Ph.D and indicates that a person who has a Ph.D. earns, on the average, $2,500 more per year than a person with the same seniority who does not have a Ph.D. Thus testing the hypothesis that β2 = 0 is equivalent to testing the hypothesis that there is no difference between the salaries of Ph.D.'s and the salaries of non-Ph.D.'s.
User Contributed Comments 3
User | Comment |
---|---|
turtle | nice ilustration, but seniority in Ph.D. should also add to wages increase :-) |
katybo | n-1 dummy variables for n categories, if not, you can't estimate regression. |
brave1986 | eally good illustration |
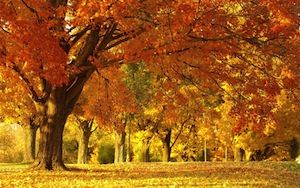
I am using your study notes and I know of at least 5 other friends of mine who used it and passed the exam last Dec. Keep up your great work!
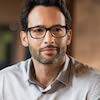
Barnes
My Own Flashcard
No flashcard found. Add a private flashcard for the subject.
Add