- CFA Exams
- 2025 Level II
- Topic 1. Quantitative Methods
- Learning Module 4. Extensions of Multiple Regression
- Subject 3. Logistic Regression Model
Seeing is believing!
Before you order, simply sign up for a free user account and in seconds you'll be experiencing the best in CFA exam preparation.
Subject 3. Logistic Regression Model PDF Download
Qualitative dependent variables are dummy variables used as dependent variables instead of as independent variables. For example, to predict whether or not a company will go bankrupt, we need to use a qualitative dependent variable (bankrupt or not) as the dependent variable.
For qualitative independent variables, analysts often utilize "dummy variables" that assume a value of one if applicable and a value of zero otherwise.
Logit models are based on the logistic distribution. Its purpose is to allow for the construction of regression equations defined by a qualitative dependent variable. The logistic transformation takes the probability that an event happens, p, divided by the probability that the event will not happen, 1 - p. The logistic transformation tends to linearize the relationship between the independent and dependent variables.
For example, a logit model may be constructed to determine the probability that a corporation defaults on its debt given values for the independent variable(s). The resulting output will be a simple probability, which is bound by zero (no probability of a debt default) and one (one-hundred percent probability of a debt default).
Logistic regression is often used in binary classification problems, which are common in machine learning and neural networks.
Logistic regression coefficients are typically estimated using the maximum likelihood estimation (MLE) method.
Interpreting Logistic Regression Models
In a logit model, the slope coefficient is the change in the logit that the event happens per unit change in the independent variable. The exponent of the slope coefficient is the ratio of odds that the event will happen with a unit increase in the independent variable.
For a logit regression, the test of the hypothesis regression coefficient is significantly different from zero; it is the same as that of an ordinary linear regression. The overall performance of a logit regression can be evaluated by examining the likelihood chi-square test statistic.
Since logistic regression cannot be fitted using at least-square approach, logistic regression has no equivalent measure for the coefficient of determination. Researchers have proposed Pseudo - R2 to compare different specifications of the same model. However, it is unsuitable when comparing models with different datasets.
User Contributed Comments 1
User | Comment |
---|---|
danlan2 | Logit model: probability, logistic distribution; probit model: similar, standard normal distribution. |
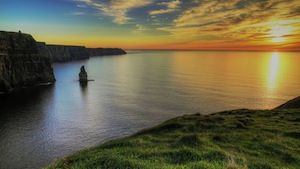
You have a wonderful website and definitely should take some credit for your members' outstanding grades.
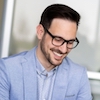
Colin Sampaleanu
My Own Flashcard
No flashcard found. Add a private flashcard for the subject.
Add