- CFA Exams
- 2025 Level II
- Topic 1. Quantitative Methods
- Learning Module 1. Basics of Multiple Regression and Underlying Assumptions
- Subject 1. A Review of Multiple Linear Regression's Uses
Why should I choose AnalystNotes?
AnalystNotes specializes in helping candidates pass. Period.
Subject 1. A Review of Multiple Linear Regression's Uses PDF Download
In financial analysis, we often need to determine the effect of more than one independent variable on a particular dependent variable. Multiple regression is a generalization of the simple linear regression analysis covered in level 1. The same ideas can be extended to analyze relationships between a dependent variable and two or more explanatory variables. If knowledge of one variable X helps us predict the value of Y, then it is natural to consider whether knowledge of several variables X1, X2, ..., Xt enables us to provide an even better prediction of the value of Y.
where
- t = 1, 2, 3, ..., T observations.
- Yt = the dependent variable.
- Xj = the independent variables, j = 1, 2, ..., k.
- Xjt = the t-th observation on the independent variable Xj.
- β0 = the intercept of the equation.
- β1, ..., βk = the slope coefficients for each of the independent variables. βj measures how much the dependent variable changes when the independent variable, Xjt, changes by one unit, holding all other independent variables constant.
- et = the error term. for any values of the independent variables, the mean value of et is 0.
We refer to both the intercept β0, and the slope coefficients, β1, ..., βk, as regression coefficients.
Analysts can use multiple regression to test existing forecasting theories. Multiple regression can further be used to identify relationships between variables after the model is tested and deemed acceptable for out-of-sample performance. For example, an analyst seeking to determine how a company's stock price and trading volume change daily can correlate them. An analyst can use linear regression to determine the relationship between variables.
A single factor cannot adequately explain or forecast the complex world of investments. Due to their complexity, statistical tests and fundamental justification are critical in the exhaustive explanation of financial and economic relations. For example, a company's profitability, growth, revenue, and market share are variables that an investor is interested in. These variables can predict if a company will run into financial difficulties.
The regression process covers several decisions the analyst must make, such as identifying the dependent and independent variables, selecting the appropriate regression model, testing if the assumptions behind linear regression are satisfied, examining goodness of fit, and making needed adjustments.
Note that regressions never identify causal relationships, only correlations (however strong they are). Parameter values often drift over time.
User Contributed Comments 0
You need to log in first to add your comment.
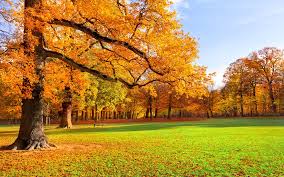
I just wanted to share the good news that I passed CFA Level I!!! Thank you for your help - I think the online question bank helped cut the clutter and made a positive difference.
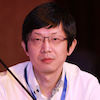
Edward Liu
My Own Flashcard
No flashcard found. Add a private flashcard for the subject.
Add