- CFA Exams
- 2025 Level II
- Topic 1. Quantitative Methods
- Learning Module 3. Model Misspecification
- Subject 3. Serial Correlation
Why should I choose AnalystNotes?
AnalystNotes specializes in helping candidates pass. Period.
Subject 3. Serial Correlation PDF Download
Serial correlation occurs when the regression residuals are correlated across observations. The problem arises when we are studying time series data. For example, data concerning annual savings and annual income for one family for 10 consecutive years are time series data. With time series data, the order in which the observations are made is important. Serially correlated errors can occur in time series studies because random events that influence Y in period (t - 1) can have lingering effects that influence Y in the following period t. If this is the case, we say that the random disturbances ei-1 and ei are serially correlated.
With time series data, however, successive errors often tend to be positively correlated. That is, positive errors tend to be followed by positive errors, and negative errors tend to be followed by negative errors, because random events that cause positive errors in one period have lasting effects that also cause positive errors in the next period.
Although the estimated parameters may be accurate, the standard errors for the coefficients are affected by positive serial correlation. Typically, positive serial correlation causes OLS standard errors for the coefficients to underestimate the true standard errors. As a consequence, if positive serial correlation is present in the regression, standard linear regression analysis will lead us to compute artificially small standard errors for the regression parameters. These small standard errors will cause the estimated t-statistics to be inflated, suggesting significance where perhaps there is none. The inflated t-statistics may, in turn, lead us to incorrectly reject null hypotheses about parameters of the regression model more often than we would if the standard errors were correctly estimated. This type I error could lead to improper investment recommendations.
Testing for Serial Correlation
To check for autocorrelation, we can calculate Durbin-Watson (DW) statistics. The value must be between 0 and 4. If DW = 2 implies no autocorrelation, 0 < DW < 2 implies positive autocorrelation, while 2 < DW < 4 indicates negative autocorrelation. The residual values can also be plotted against time to identify seasonal or correlated patterns.
The Breusch-Godfrey (BG) test is a robust method for detecting serial correlation. The BG test uses residuals from the original regression as the dependent variable run against initial regressors plus lagged residuals, and H0 is the coefficients of the lagged residuals are zero.
While the DB test is aimed at an autocorrelation of 1st order, the BG test can also uncover autocorrelation of higher orders.
Correcting Autocorrelation
There are two methods to reduce or eliminate serial correlation bias.
- First, the standard errors of the regression coefficients can be adjusted to reflect the serial correlation. The resulting standard errors are referred to as "robust standard errors." Hansen's method, which can be found in many statistical software packages, is the most prevalent method for adjusting standard errors.
- The second method involves a reconfiguration of the equation defining the regression. By utilizing this correction method, the regression equation is modified in an attempt to mitigate the autocorrelation bias. This course of action, i.e., reconfiguring the regression equation, should be taken with extreme care, as slight miscalculations can result in invalid estimates for the underlying regression parameters.
- When encountered by a regression suffering from serial correlation bias, analysts are encouraged to correct for the autocorrelation by adjusting the coefficient standard errors to account for the serial correlation.
User Contributed Comments 4
User | Comment |
---|---|
danlan2 | Two methods: robust standard errors and reconfiguration, similar to heteroskedasticity. |
REITboy | D-W Statistic = (Sum of squared differences between sequential residuals) OVER (Sum of squared residuals) If n is large, DW Stat is approximated by: DW = 2(1-r) where r = correlation coefficient between sequential squared residuals DW < 2 implies positive serial correlation DW = 2 implies no serial correlation DW > 2 implies negative serial correlation |
REITboy | ...guess I should have waited for the next reading... |
philjoe | hahahaha |
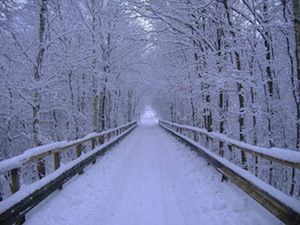
I am using your study notes and I know of at least 5 other friends of mine who used it and passed the exam last Dec. Keep up your great work!
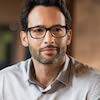
Barnes
My Own Flashcard
No flashcard found. Add a private flashcard for the subject.
Add