- CFA Exams
- 2025 Level I
- Topic 1. Quantitative Methods
- Learning Module 8. Hypothesis Testing
- Subject 6. The Decision Rule
Seeing is believing!
Before you order, simply sign up for a free user account and in seconds you'll be experiencing the best in CFA exam preparation.
Subject 6. The Decision Rule PDF Download
A decision rule is a statement of the conditions under which the null hypothesis will be rejected and under which it will not be rejected.
The critical value (or rejection point) is the dividing point between the region where the null hypothesis is rejected and the region where it is not rejected. The region of rejection defines the location of all those values that are so large (in absolute value) that the probability of their occurrence is low if the null hypothesis is true.
In general, the decision rule is that:
- If the magnitude of the calculated test statistic exceeds the rejection point(s), the result is considered statistically significant and the null hypothesis (H0) should be rejected.
- Otherwise, the result is considered not statistically significant and the null hypothesis (H0) should not be rejected.
The specific decision rule varies depending on two factors:
- The distribution of the test statistic.
- Whether the hypothesis test is one-tailed or two-tailed.
For the following discussion, you assume that the test statistic follows a standard normal distribution. Therefore:
- The calculated value of the test statistic is a standard normal variable, denoted by z.
- Rejection points are determined from the standard normal distribution (z-distribution).
The Decision Rule for a Two-Tailed Test
A two-tailed hypothesis test for the population mean (μ) is structured as H0: μ = μ0 vs. H1: μ ≠ μ0. For a given level of significance (α), the total probability of a Type I error must sum to α, with α/2 in each tail. Therefore, the decision rule for a two-tailed test is:
where zα/2 is chosen such that the probability of z > zα/2 is α/2.
For a two-sided test at the 5% significance level, the total probability of a Type I error must sum to 5%, with 5%/2 = 2.5% in each tail. As a result, the two rejection points are -z0.025 = -1.96 and z0.025 = 1.96. These values are obtained from the z-table. Therefore, the null hypothesis can be rejected if z < -1.96 or z > 1.96, where z is the calculated value of the test statistic. If the calculated value of the test statistic falls within the range of -1.96 and 1.96, the null hypothesis cannot be rejected.
The Decision Rule for a One-Tailed Test
A one-tailed hypothesis test for the population mean (μ) is structured as H0: μ ≤ μ0 vs. H1: μ > μ0. For a given level of significance (α), the probability of a Type I error equals α, all in the right tail. Therefore, the decision rule for a one-tailed test is:
where zα is chosen such that the probability of z > zα is α.
For a test of H0: μ ≤ μ0 vs. H1: μ > μ0 at the 5% significance level, the total probability of a Type I error equals 5%, all in the right tail. As a result, the rejection point is z0.05 = 1.645. Therefore, the null hypothesis will be rejected if z > 1.645, where z is the calculated value of the test statistic. If z ≤ 1.645, the null hypothesis cannot be rejected.
If a one-tailed hypothesis test for the population mean (μ) is structured as H0: μ ≥ μ0 vs. H1: μ < μ0, similar analysis can be performed (i.e., reject H0 if z < -zα).
Statistical Decision vs. Economic Decision.
Making a statistical decision involves using the stated decision rule to determine whether or not to reject the null hypothesis. A statistical decision is based solely on statistical analysis of the sample information. The economic or investment decision takes into consideration not only the statistical decision, but also all economic issues pertinent to the decision. Slight differences from a hypothesized value may be statistically significant but not economically meaningful (taking into account transaction costs, taxes, and risk).
For example, it may be that a particular strategy has been shown to be statistically significant in generating value-added returns. However, the costs of implementing that strategy may be such that the added value is not sufficient to justify the costs required. Thus, while statistical significance may suggest a particular course of action is optimal, the economic decision also takes into account the costs associated with the strategy, and whether the expected benefits justify implementing the strategy.
Researchers frequently report the p-value associated with a particular test in order to present a fuller picture.
The P-Value Approach to Hypothesis Testing
Note: This is not required by the study guide.
If you go back to subject a, you will see that seven steps in any hypothesis testing procedure are listed. These seven steps compose the process that needs to be carried out in order to perform a test.
However, there is an alternative and widely used approach to hypothesis testing, which will be discussed here. This is not a different method, but rather a different way of presenting the results of a test. The new approach is known as the p-value approach to hypothesis testing.
Before proceeding with the new approach, a summary of the seven steps encountered earlier:
Step 1: State the hypotheses.
Step 2: Identify the test statistic and its probability distribution.
Step 3: Specify the significance level.
Step 4: State the decision rule.
Step 5: Collect the data in the sample and calculate the necessary value(s) using the sample data.
Step 6: Make a decision regarding the hypotheses.
When a p-value approach is used, steps 3 and 4 become redundant. The new steps are as follows:
Step 1: State the hypotheses.
Step 2: Identify the test statistic and its probability distribution.
Step 3: Collect the data in the sample and calculate the necessary value(s) using the sample data.
Step 4: Calculate the p-value for the test.
Step 5: Make a decision regarding the hypotheses.
Step 6: Make a decision based on the test results.
It can thus be seen that the p-value approach is simpler, and also provides useful information about a test statistic. So, by now you are probably anxious to find out: What exactly is a p-value?
P-value is the area from the test statistic to the end of the tail (or tails, in the case of a two-sided test) of interest. The "p" stands for probability, and the p-value is a probability. Effectively, it represents the chance of obtaining a test statistic whose value is at least as extreme as the one just calculated in the test, when H0 is true.
The term "tail of interest" means the right-hand tail in a > test, the left-hand tail in a < test, and both tails in a ≠ test.
It should be clear to you that if the test statistic is very extreme (that is, close to the tail) then the area from that value to the end of the tail will be very small. By definition, the p-value is thus very small. In this case, the test statistic would certainly fall in the rejection region.
Conversely, if the test statistic is not extreme (that is, it is far from the tail) and would thus fall in the acceptance region, the area from the test statistic to the end of the tail would be fairly large. In this case, the p-value is fairly large.
So, you have just learned how to interpret p-values. A small p-value indicates a rejection of the null hypothesis whereas a large p-value indicates a non-rejection of the null hypothesis.
This is all well and good, but how large is large?
P-value is normally compared with our usual α value, so 0.05 is a common reference point. This means that, for p-values smaller than 0.05, H0 would be rejected, and for p-values larger than 0.05, H0 would not be rejected.
However, p-value provides not only a reference point but an idea about just how strong or weak this rejection or non-rejection is.
For example, a p-value of 0.0003 would indicate a very strong rejection of H0 at virtually any a-level, whereas a p-value of 0.048 would indicate a rejection of H0 when compared with a value of 0.05 but a non-rejection of H0 when compared with an α value of 0.01.
In general, the larger the p-value, the smaller the likelihood that H0 will be rejected and vice versa.
In the case of a two-sided test, if the area from the test statistic to the end of the right tail is 0.04, for example, then the p-value will be 0.08; doubling the area is necessary because both tails are being used. It's as simple as that.
Examples
Now try the following for yourself. In each case, determine whether or not you would reject H0 when compared with an α value of 0.05.
You are given the following p-values. A. 0.02
B. 0.456
C. 0.053
D. 0.0001
The correct answers are:
A. Reject H0.
B. Do not reject H0: The p-value is very large (over 45%), and therefore you are very far away from a rejection of H0.
C. Do not reject H0: The p-value is only just more than 0.05, so you are close to a rejection.
D. Reject H0: The p-value is very small, so you would reject H0 in almost all cases.
Now that you understand how p-values work, let us go through the testing procedure again:
- First write down your hypotheses, then determine which test statistic to use. There is no need to have a significance level, and hence, you don't have a specifically demarcated rejection region.
- Calculate the test statistic and determine the resultant p-value.
- The conclusion of the test is based on your p-value. Recall that a small p-value means a rejection of H0, whereas a large p-value means a non-rejection of H0.
Note: P-values can be worked out for all continuous statistical distributions, no matter what the shape of the distribution is. So, this method can be used for z-graphs, t-graphs, x2-graphs and F-graphs. You will encounter the x2- and F-distributions later on in this chapter.
Confidence Interval and Hypothesis Testing
A confidence interval is a range of values within which it is believed that a certain unknown population parameter (often the mean) will fall with a certain degree of confidence. The percentage of confidence is denoted (1 - α)%.
A test of significance is a test to ascertain whether or not the value of an unknown population parameter is as stated by an individual or institution. This test is carried out at a significance level of α, which determines the size of the rejection region.
Note how the confidence interval is related to the test statistic. They are linked by the rejection point(s).
- The confidence interval is:
- Recall that the test statistic is:
The likelihood that the z-value will be less than the test statistic is what is being tested. Setting up this inequality, and rearranging, the test is:
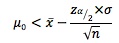
Confidence intervals can be used to test hypotheses. Note that the right side of the equation is the left endpoint of the confidence interval. Essentially, if the confidence interval contains the value of the unknown population parameter as hypothesized under H0, then H0 would not be rejected in a two-sided hypothesis test with corresponding α; if the confidence interval does not contain the value of the unknown population parameter as hypothesized under H0, then H0 would be rejected in a two-sided hypothesis test with corresponding α.
The reason for the above determination is as follows:
- If the hypothesized value does not fall in the confidence interval, then there is a very small chance that the value can be a true value for the unknown parameter, so H0 is almost certainly wrong and will be rejected.
- If the hypothesized value does fall in the confidence interval, then there is a very good chance that the value can be a true value for the unknown parameter, so H0 is definitely possible and will not be rejected.
This comparison can be used only for two-sided tests, not one-sided tests, because confidence intervals cannot be linked with one-sided tests.
User Contributed Comments 4
User | Comment |
---|---|
gill15 | Notes here need to be condensed to p value less then z ---- reject null |
sharky7 | *Decision rule |
bschmitt | it is written here that the p-value is not required by the study guide, but I find it in the study guide and I do not see it would not be required. can anyone help on that please? |
mcbreatz | The P value is about 3 paragraphs in the text and it is pretty much explained exactly as above where smaller than critical value will reject null. Might possibly be 1 question on test. |
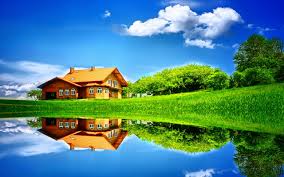
I passed! I did not get a chance to tell you before the exam - but your site was excellent. I will definitely take it next year for Level II.
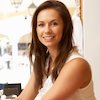
Tamara Schultz
My Own Flashcard
No flashcard found. Add a private flashcard for the subject.
Add